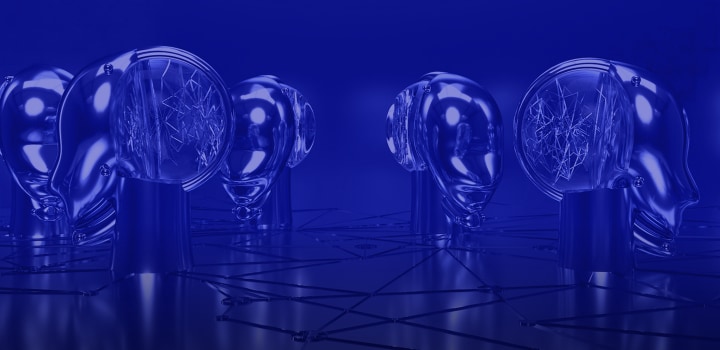
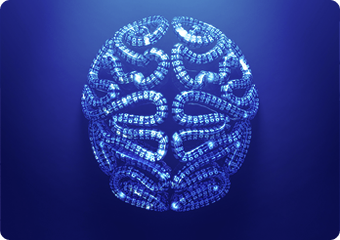
Generative AI is the latest buzz in the tech world. They have taken the world by storm and amassed a huge fan following, much like a cult!. But did you know their true enterprise potential is still limited to task-specific applications? The current models are only trained on external, publicly available datasets and cannot access proprietary enterprise data. That’s where the real value of generative AI lies – when businesses can use it as part of their day-to-day workflows and business processes in a secure, risk-free, and unbiased way. And for that, enterprises need a bridge between their workflows and the capabilities of generative AI. If you’re an enterprise looking to unlock the full potential of generative AI, read on to find out more about the solutions available.
The hype around ChatGPT since its launch in November 2022 put the spotlight on Generative AI. Garnering a million users in just five days of launch, ChatGPT showcased the potential and demand for AI models that could help people augment their capabilities. From a consumer perspective, we’ve all heard the news about how people successfully use it to create art, content, code, and even launch and run businesses. And it’s not ChatGPT alone; a spurt of generative AI models has found its way into common parlance. But what does generative AI mean for the enterprise? And how can businesses use it to unleash its full potential?
ChatGPT is an application sitting on top of different GPT models. When the first GPT model came out in 2018, while significantly powerful, it was not easy to use. This complexity made it difficult for people to work with it. And so, the model stayed in the realm of complex engineering or high-end data science efforts. The introduction of ChatGPT democratized generative AI. This application made it simple for anyone to benefit from a large language model with conversations that were magnitude order more reliable, human-like, relevant, and high-quality. This application could correct itself, apologize and admit it’s wrong – and then give a better answer. It could sustain a whole conversation lifecycle – fully understanding where you started and where you are in that conversation and giving progressively contextual answers. This approach phenomenally changed how people saw generative AI’s benefits and made it a household name.
The Challenge of Using Generative AI For the Enterprise
While there is nothing stopping enterprise users from using models like ChatGPT to support their work – for instance, to get more contextual answers to their queries or use it embedded in Microsoft applications such as Sharepoint, Edge browser, and Bing – the true potential of generative AI is lost to them. These avenues of exploring generative AI limit it to a task-specific application.
An enterprise workflow is significantly more complex, with legacy processes supported by many applications. By nature, these systems are in siloes. In addition, the existing Generative Pre-trained Transformers (GPT) and Large Language Models (LLM) are trained on external, publicly available data sets and cannot access proprietary enterprise data. And so, they are not equipped to answer queries based on internal data such as, “What is the revenue for the XYZ product in Germany?” Or “Who are the key people within my organization who can support ABC project?”
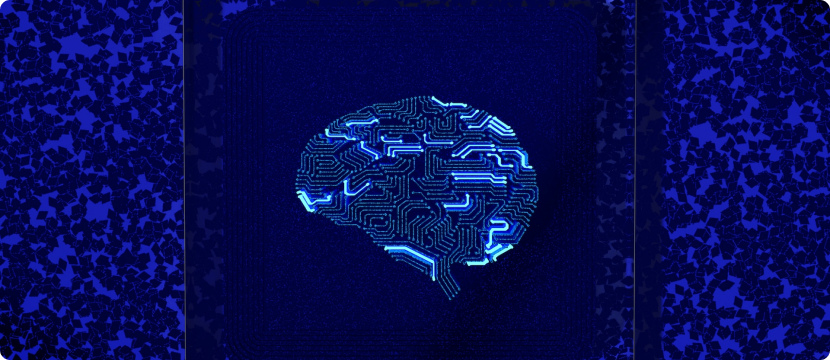
Generative AI Isn’t New in the Enterprise
While the hype is new, generative AI models have been around for some time (See Fig 1), and enterprises have been using internal data to build their models. In fact, for the last five years, EdgeVerve has been at the forefront of using Transformer and CNN models for NLP and computer vision use cases for our clients (See Fig 2). Leading analysts and industry bodies have recognized our work and capabilities.
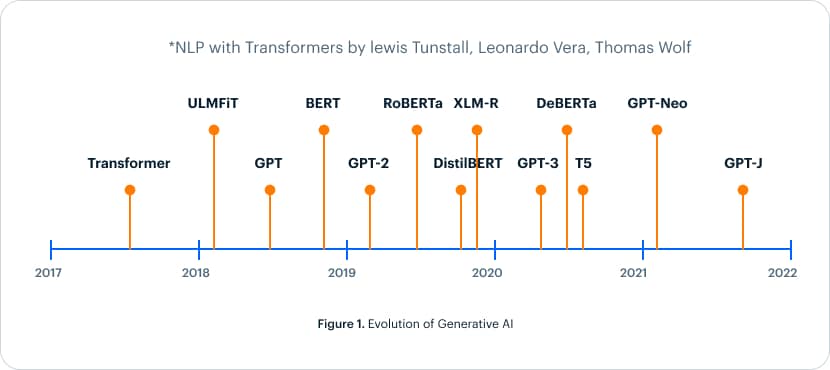
We have worked with our customers to enable these capabilities in their businesses and delivered tremendous value. For instance:
For one of the largest telecommunications companies in the world, we used deep learning models based on transformers and large language models to help improve employee productivity by 60% and saved USD 20 million. We made the information available at the client’s fingertips by identifying, extracting, and managing data from over 650,000+ historic commercial tower lease contracts – with many amendments, addendums, and related documents. The client’s teams could navigate through 650K+ contracts instantly and filter them using query-based and logical operators for identification as per specific keyword and phrase combinations.
#2 – Simplifying medical literature writing
We helped a healthcare client use generative models to summarize manuscripts for
medical literature. Medical research and publishing require a lot of referencing and citation of existing work. Done manually, it’s a tedious and complex process that demands a significant amount of time investment from high-end experts such as scientists, researchers, doctors, etc. Using large language models, we automated the creation of the first draft of the paper, complete with relevant citations saving significant time and effort that is better used elsewhere.
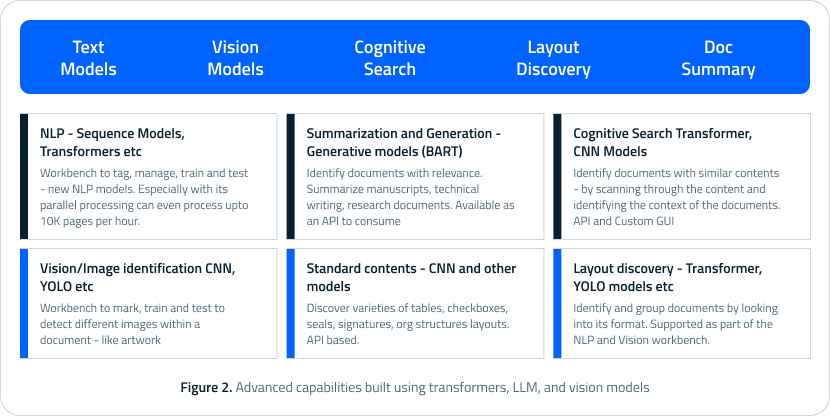
In another instance, we enabled a cognitive search for a leading manufacturer of commercial trucks based in the US. The company heavily customized its vehicles, making servicing and repair challenging and time-consuming. Every time a car rolled in for repair, the service staff spent two to three weeks figuring out what went into the truck and trying to correlate the issue to some defect in a component to either change it or service it. This meant reviewing manuals, guides, and documents related to that specific truck. We used transformers and LLMs to extract information from these documents and make them indexed and searchable. Now the support staff can describe the problem, and the system extracts the correct answers for them in no time at all!
#4 – Document discovery to create a training corpus
We helped a US-based financial services company providing clearing and settlement services extract information from complex and voluminous legal documents such as a bond prospectus. We used large language models to narrow down relevant documents from a historic set of thousands of documents, each containing hundreds of pages of legalese.
While these use cases solve some critical challenges for enterprises, their outcomes can be enriched manifold by integrating with GPT models.
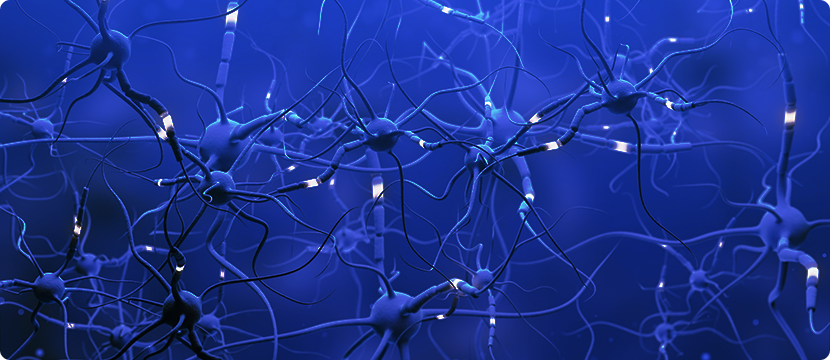
Integrating GPT Models with Enterprise Data
As mentioned earlier, the value of generative AI in the enterprise will unlock when they can use it as part of their day-to-day workflows and business processes in a secure, risk-free, and unbiased way. Enterprises need a bridge between their workflows and GPT capabilities to enable this scenario.
However, as we explore the immense possibilities of GPT, we should also be conscious of the risks. While these models are well-trained on humongous data sets, multi-modal, and can reason like humans, they are still volatile. They are prone to jailbreaks, and the controls put in place to ensure that the AI responses are sanitized, and bias-free can be overridden. This is a problematic situation; for example, a chatbot built on GPT may not adhere to enterprise standards of acceptable responses – when interacting with a customer, this could pose a reputation risk.
And finally, the GPT models are dated and can only provide information till the point in time when they were last trained. GPT4, for instance, was last trained in September 2021, and its responses are limited to information before that time frame.
Loved what you read?
Get practical thought leadership articles on AI and Automation delivered to your inbox
Loved what you read?
Get practical thought leadership articles on AI and Automation delivered to your inbox
A Glimpse into the Future
In a recent research report, OpenAI stated that 49% of workers could have half or more of their tasks exposed to LLMs1. Interestingly, unlike other technologies, this impact is much higher for knowledge workers. How can you compete with a system that can access humanity’s collective knowledge in seconds? While the business impact of technology might be exponential, we need to start thinking about what it means for the economy and the future of the workforce. As enterprises adopt these new generative models, they need to weigh this technology’s risks, rewards, and broader impact and create an ecosystem that addresses these issues upfront.
Disclaimer Any opinions, findings, and conclusions or recommendations expressed in this material are those of the author(s) and do not necessarily reflect the views of the respective institutions or funding agencies
References: